What is Data Labeling?
Posted 2024-05-09 11:31:02
0
350
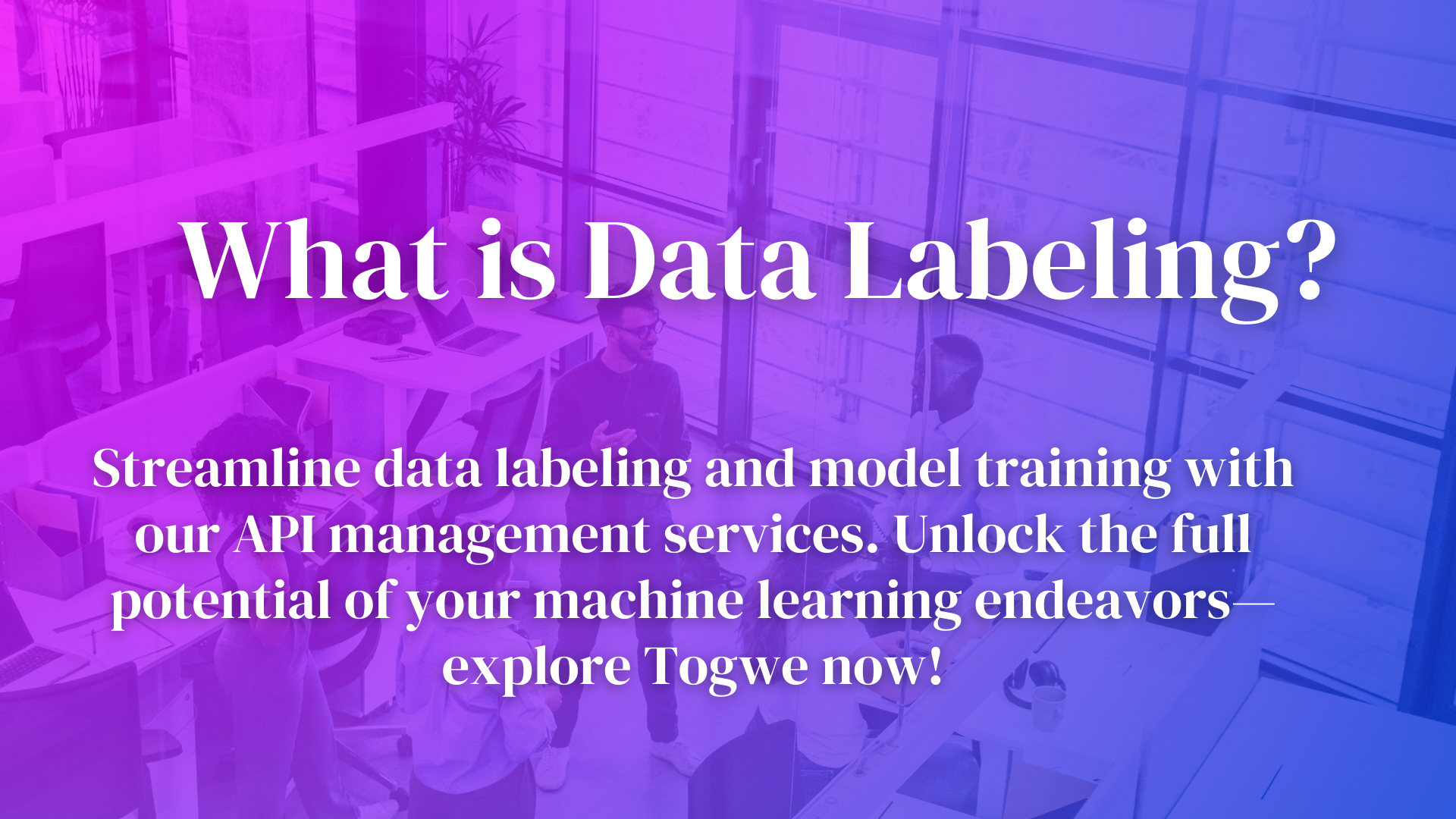
Discover the importance, methodologies, challenges, and best practices of data labeling in machine learning models. Learn how API management services like Togwe enhance the data annotation process.
Data labeling plays a crucial role in the development of machine learning models, providing the necessary context for accurate predictions. This practice involves tagging raw data, such as images and text files, to facilitate the training and validation of AI algorithms. In this blog, we’ll delve into the importance of data labeling, its methodologies, challenges, best practices, applications, and how API management serviceslike Togwe can enhance the data annotation process.
Why is Data Labeling Important?
Data labelingserves as the foundation for various machine learning and deep learning applications, including computer vision and natural language processing. Through the assignment of labels to datasets, analysts can effectively isolate variables, facilitating the identification of the best predictors for model training. Human-in-the-loop (HITL) involvement is essential in guiding the data labeling process, actively contributing to the development, training, refinement, and evaluation of ML models.
Labeled Data vs. Unlabeled Data:
Understanding the distinction between labeled and unlabeled data is important. Labeled data is employed in supervised learning, requiring time and resources for accurate annotations. Unlabeled data, on the other hand, is more accessible but limited in its usefulness. Combining both types for semi-supervised learning reduces the need for extensive manual labeling while providing a substantial annotated dataset.
Challenges in Data Labeling:
-
Workforce Management: Skilled Team Dynamics are crucial in data labeling, requiring a proficient team of data scientists and engineers for high-quality work. Quality Assurance involves overcoming cultural and language barriers, ensuring data precision, and validation.
-
Data Oversight: Subjectivity challenges arise from personal biases and cultural nuances within the team. Objectivity concerns stem from data illiteracy, potentially leading to inaccurate data labels and AI misinterpretations.
-
Optimal Tool Selection: Judicious choices in software and skilled labelers are paramount for a successful ML process. Awareness and utilization of open-source tools for data labeling are indispensable to meet specific data requirements.
-
Cost-Effective Implementation: In-House Tool Development can be advantageous but may escalate costs and impede market deployment timelines. A lack of transparency in the data labeling process can hinder funding and project sponsorship, creating obstacles for cost-effective implementation.
-
Adhering to Security Standards: Compliance challenges involve conforming to global data security standards like GDPR and CCPA, imperative for data labeling companies.
Benefits of Data Labeling:
-
Consistent Data Output: Reliability in consistent data output is achieved through in-house tools, ensuring accuracy and long-term dependability.
-
Iterative Feedback System: Continuous performance enhancement is achieved through an annotation feedback loop, actively refining the labeling process over time.
-
Automated Labeling Processes: Automation contributes to efficiency and precision, minimizing costs and expediting the labeling process.
-
Proficiency in Deep Learning: Deep learning coupled with ML algorithms efficiently handles unstructured data, ensuring accurate outcomes.
Best Practices in Data Labeling:
-
Collect Diverse Data
-
Set Clear Annotation Guidelines
-
Implement Quality Assurance
-
Choose an Effective Annotation Pipeline
-
Maintain Open Communication
-
Run Pilot Projects
Applications of Data Labeling:
-
Training Data for Machine Learning Models
-
Evaluation and Validation of Models
-
Active Learning and Semi-Supervised Learning
-
Data Pre-processing and Augmentation
-
Anomaly Detection and Fraud Prevention
-
Natural Language Processing (NLP) Tasks
-
Computer Vision Applications
In conclusion, data labelingis a pivotal force shaping the accuracy and efficiency of machine learning models. From understanding its importance to navigating challenges and adopting best practices, strategic investments in data labeling are indispensable for companies aspiring to harness the economic advantages of cutting-edge technologies. With the integration of API management services like Togwe, the data annotation process can be further optimized, revolutionizing AI solutions and unlocking their full potential.
Site içinde arama yapın
Kategoriler
- Art
- Causes
- Crafts
- Dance
- Drinks
- Film
- Fitness
- Food
- Oyunlar
- Gardening
- Health
- Home
- Literature
- Music
- Networking
- Other
- Party
- Religion
- Shopping
- Sports
- Theater
- Wellness
Read More
Hoodie Trends 2024: What's Hot in Essential Streetwear
As we step into 2024, the world of streetwear continues to evolve, with hoodies remaining at the...
Chromatography Resins Procurement Intelligence Develop at a CAGR of 6.9% Till 2030
Chromatography Resins Procurement Intelligence
The chromatography resins category is...
Cannaleafz CBD Gummies Canada- Reduces Fatigue & Keeps You Energetic & Active!
Cannaleafz CBD Gummies Canada Review:- Millions of individuals all over the planet grumble about...
Miami Web Design Company: Convert Website Visitors into Sales
Conversions & Customer Engagement: WebCreationDesign, a Miami web design company, crafts...
https://www.facebook.com/MaxKetosisKetoACVGummiesOfficial/
👇👇Official Website -- Click Here To-- Get More Discount👇👇
⚠️Beware of Fake Websites⚠️
➢Item...